Thales unit develops metamodel to detect deepfake images
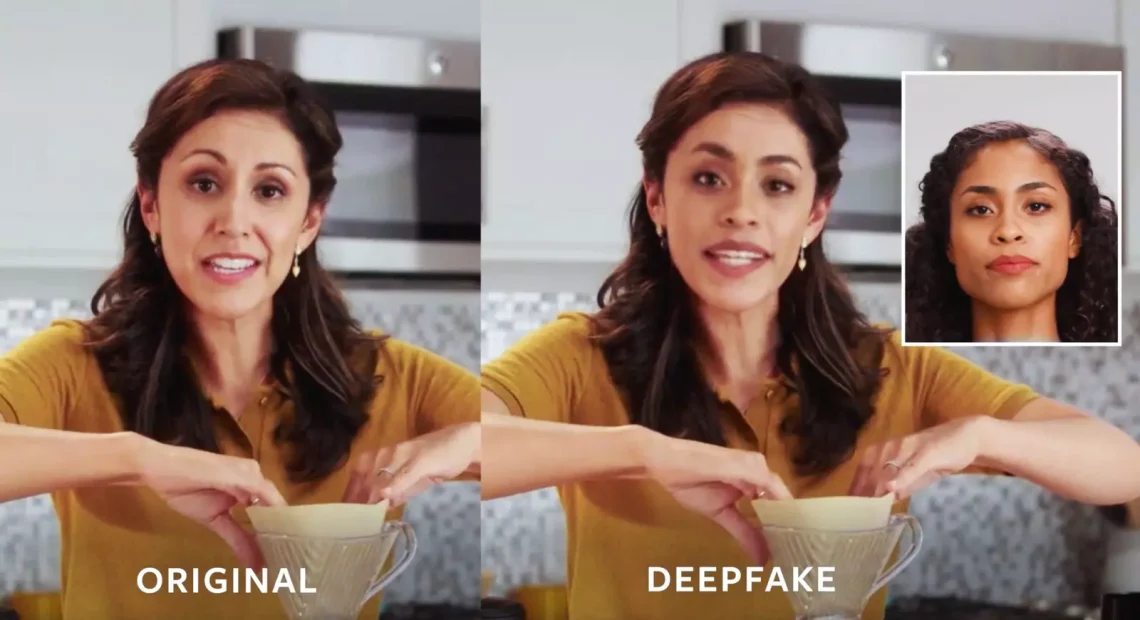
Deepfake images are a growing problem
As artificial intelligence (AI) becomes more sophisticated, so do the risks it brings, especially regarding disinformation and digital manipulation. One of the most concerning manifestations of AI is the creation of deepfake images, videos, and audio that can be used to deceive and defraud.
On November 20, 2024, Thales’s Friendly Hackers unit, known as cortAIx, introduced an innovative solution to this growing problem by developing a metamodel to detect AI-generated deepfakes.
Deepfakes are no longer a rarity. With platforms like Midjourney, Dall-E, and Firefly, creating hyper-realistic images, videos, and audio has become more accessible. While these technologies have vast potential for creativity and innovation, they are equally ripe for exploitation, especially in areas like identity theft, fraud, and malicious disinformation campaigns.
Deepfake technology has raised alarms across multiple industries, from media to cybersecurity, as it becomes increasingly difficult to distinguish between real and fake content.
Thales, a global technology leader, has been at the forefront of developing solutions to counter AI’s adverse side effects. Their recent innovation, a metamodel for detecting deepfakes, is a crucial step forward in combating the malicious use of AI-generated content.
The breakthrough was developed as part of a challenge initiated by France’s Defence Innovation Agency (AID), coinciding with the European Cyber Week event held in Rennes, Brittany.
What is a metamodel?
At its core, a metamodel is a model that combines multiple models to improve the accuracy and reliability of results. In Thales’s case, the metamodel aggregates different deepfake detection methods to enhance the identification of AI-generated content. Each model assigns an authenticity score to an image, helping to determine whether it is real or fake based on various factors like noise patterns and visual inconsistencies.
The Thales deepfake detection metamodel uses machine learning algorithms, decision trees, and advanced analytics to assess an image’s authenticity. The metamodel can more effectively identify deepfakes and flag potentially fraudulent content by evaluating strengths and weaknesses across several detection techniques.
What makes this metamodel genuinely innovative is the use of multiple detection methods. Each method focuses on a specific aspect of an image, such as visual artefacts or inconsistencies between text and visuals, to identify deepfakes. This multi-pronged approach improves the chances of catching even the most sophisticated AI-generated images.
The CLIP method, which stands for Contrastive Language-Image Pre-training, is one of the core techniques in the Thales metamodel. It connects images with textual descriptions and compares them to detect inconsistencies. By learning how language and images correlate, CLIP can spot discrepancies between an image and its associated text, often a telltale sign of deepfake manipulation.
The DNF (Diffusion Noise Feature) method identifies the noise patterns characteristic of AI-generated images. Diffusion models, commonly used in AI image generation, introduce noise to create new content. The DNF method can pinpoint when an image has been artificially generated by analysing these noise patterns.
Different approach
The DCT (Discrete Cosine Transform) method takes a different approach by examining an image’s frequency domain. It looks for subtle anomalies in the structure of an image that can’t be seen with the naked eye. These anomalies often arise during deepfake creation and can be detected using DCT, even if hidden in the pixels.
The development of the deepfake detection metamodel wasn’t an overnight success. It relies on various cutting-edge technologies and techniques honed over years of research.
Machine learning is the backbone of the metamodel, allowing it to learn from vast datasets of authentic and fake images. Through decision trees, the model evaluates different characteristics of an image to assign an authenticity score. Over time, the model improves accuracy by analysing more data and learning from past predictions.
Noise detection and spatial frequency analysis are critical components in identifying AI-generated deepfakes. These techniques help uncover hidden artefacts and irregularities often present in manipulated images but not immediately visible to the human eye.
The implications of deepfake technology extend far beyond social media. Deepfakes can be used for identity theft, fraud, and even political manipulation. Thales’s metamodel provides a powerful tool to fight these threats.
One of the most pressing applications of deepfake detection is in the fight against identity fraud. By detecting manipulated images, Thales’s technology can prevent fake identities in various contexts, from banking to online security.
Biometric security, such as facial recognition and fingerprint scanning, is increasingly used to verify identity. However, deepfakes pose a significant risk to these systems. Thales’s metamodel offers a way to protect against this risk, ensuring that biometric checks remain secure.
With the growing use of AI in cyberattacks and cyber defence, it’s crucial to develop robust systems capable of detecting and countering AI-driven threats. Thales’s deepfake detection technology is an essential part of this effort.
AI-generated deepfakes have become a weapon in disinformation campaigns, making distinguishing between truth and fiction harder. These campaigns can have wide-reaching consequences, from influencing elections to manipulating stock markets.
The rise of deepfakes also presents a serious threat to financial security. Studies have shown that deepfakes are increasingly being used in fraud schemes, such as advanced phishing attacks, to manipulate victims into revealing sensitive financial information.
Thales’s AI accelerator, cortAIx, is home to a team of over 600 researchers and engineers dedicated to pushing the boundaries of AI technology. This team’s expertise in mission-critical systems has been instrumental in developing the deepfake detection metamodel.
The cortAIx team includes some of the brightest minds in AI research. It is based at Thales’s Saclay research facility near Paris. Their work on deepfake detection is a testament to their commitment to advancing AI in ways that protect society.
Hero image: Example of a deepfake image: original on the left, altered deepfake on the right. Credit: Witness Media Lab/WEF